class: center, middle, inverse, title-slide # Analysis Reproducibility ## Why it matters and how to do it? ### ### 8 July 2021 --- layout: true <div class="my-footer"><span></span></div> --- # Learning objectives **About today:** - Understand what you can gain from analysis reproducibility. - Know what the main technical requirements are to set up for their analysis to be reproducible. - Have a demonstration of a practical way to make a cake using household survey data: crunching, analysis & interpretation & data stories! **Not today:** - Induction Training on R language! For this head to [UNHCR Learn & Connect- R training](https://unhcr.csod.com/ui/lms-learner-playlist/PlaylistDetails?playlistId=e90e2279-e3a4-4ef2-8b74-757f91d224b2) ??? Have you ever completed the development of a data product with the feeling that you may have done a mistake or not have used the optimal way to clean or process your data? As an analyst, if using “point & click” interface, “rewinding” all the steps at an advanced stage of the development of your product can be extremely painful and lengthy. Errare humanum est sed perseverare... If you want to learn from your mistake rather than to suffer from them, then analysis reproducibility is what you need... In this session, we will introduce you to the basics of analysis reproducibility and explain you what elements you need to watch for when you kick start your analysis so that you can always rewind and improve any products you have already spent time on. We will also show you how you can learn from analysis done in a reproducible way done by other colleagues. We will also show you through practical examples how to implement a fully reproducible data analysis workflow applied to a Household Survey dataset using R statistical Language: from initial data exploration to joint interpretation till the creation of data stories. Last, we hope that this session will motivate you to join the vibrant R users community in UNHCR and soon become an R champion. In order to make the most of the session, we would advise you to install the following open source environment: R - https://cran.r-project.org/bin/windows/base/ Rstudio Free version: https://www.rstudio.com/products/rstudio/download/ Create an account on Github - https://github.com/join? and install Github desktop https://desktop.github.com/ You may also start installing UNHCR Packages – following the instruction in their respective documentation published on Github: Use UNHCR Open data - https://unhcr.github.io/unhcrdatapackage/docs/ API to connect to internal data source - https://unhcr-web.github.io/hcrdata/docs/ Perform High Frequency Check https://unhcr.github.io/HighFrequencyChecks/docs/ Process data crunching for survey dataset - https://unhcr.github.io/koboloadeR/docs/ Use UNHCR graphical template- https://unhcr-web.github.io/unhcRstyle/docs/ Last, you may also take advantage of going through one or more of the R learning content on Learn & Connect: Achieve your potential: UNHCR (csod.com) and see some practical tutorial on https://humanitarian-user-group.github.io/ The best way to start and learn is to have a concrete project! If you have one and need mentoring, we can liaise after the session. --- ## A Vision for data analysis <span style='font-size:50px; color:grey;'>"Multi-functional teams, with strengthened data literacy, regularly conduct meaningful and documented joint data interpretation sessions to define their strategic directions based on statistical evidences"</span> --- ## A Theory of Change for Data analysis <span style='font-size:30px;'>Proper user of data for advocacy & programmatic decision making </span> <span style='font-size:40px;'>↪</span> Corporate __Standards__ exist to define how to encode & process household surveys dataset <span style='font-size:40px;'>↪</span> Field data experts are trained based on precise recipes and predefined tools at each step of the __data life cycle__ <span style='font-size:40px;'>↪</span> Data are presented, discussed and linked to expert knowledge during data __interpretation__ sessions with a multi-functional team <span style='font-size:40px;'>↪</span> All potential valid interpretations, including diverging views, are systematically __recorded__ <span style='font-size:40px;'>↪</span> __Persuasive__ "Data Stories“ and Policy papers are generated --- ## Data Science is like cooking .pull-left[ When a chef is starting out with a new dish... - Hypothesis Tasting -- Setting the right questions - Ingredients = source the Data - Wash your food = clean your data - Flavor engineering = create calculated & derived variables - Taste and explore = reshape & visualize the data - Tune your oven = statistical modeling - Art of plating = use styled brand - Document your recipe = add technical comments ] .pull-right[ .img85[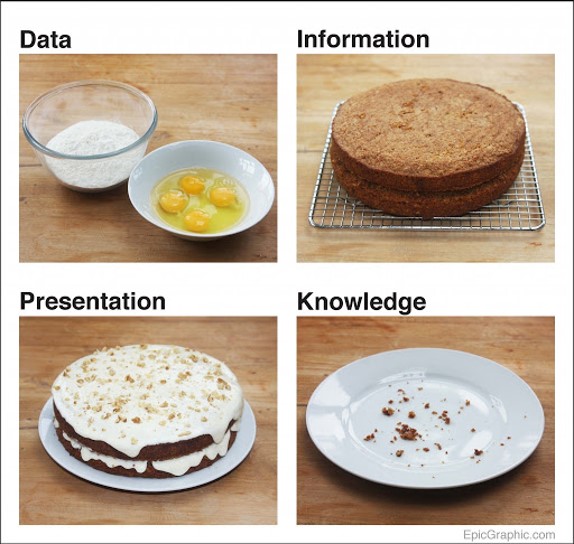] ] ??? https://towardsdatascience.com/data-science-explained-with-cooking-1a801731d749 https://towardsdatascience.com/5-reasons-why-data-science-is-like-cooking-daa506b4166a Without good ingredients, you can’t cook a good dish. Most time and effort are spent on cleaning and preparing the ingredients. Different tools and techniques are needed for different recipes. Cooking is both a science and an art. You can’t become a great cook overnight. --- ## Information Anxiety & Analysis paralysis <span style='font-size:30px;'> _When people do not want to eat the cake..._ </span> .pull-left[ Potential source of reluctance... * I do not know how to eat it: I see all those elements on it without being able to understand why they were added there and how this works... * I do not trust this cake: How was it created? Did you follow correctly the recipe? Were the ingredient fresh? Can I trust how you sourced the ingredient? * This is not the cake I need! It looks too heavy & too big: I will not be able to digest it... * I am not hungry and do not even know what cake I want... ] .pull-right[ .img[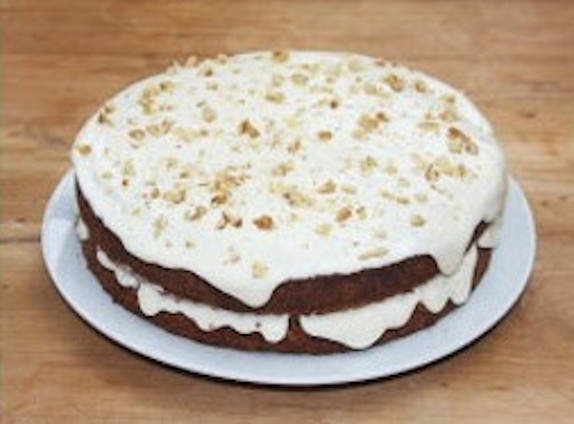] ] ??? simple data visualization for decision making or complex patterns interpretation for knowledge building --- .pull-left[ ## Data Products: When What? <span style='font-size:30px;'> Dashboard are relevant for displaying KPIs! (_like when you drive your car..._)</span> > Key Performance Indicators (KPIs) are indicators specificlly designed to show progress toward an intended result, i.e a predefined __target__ > Create an analytical basis for __decision making__, aka Business Intelligence > Help focus attention of Snr Management on what matters most - a good dashbaord needs to be __concise__ ] .pull-right[
] ??? --- class: center, middle, inverse # Why we need to work in a reproducible way? ### Ethics, Productivity, Learning --- ## Ethics: Science is '_show me_' - not '_trust me_' .pull-left[ <span style='font-size:30px;'>Reproducibility allows for __peer review__</span> Peer Review allows for __transparency__ Transparency allows for __scrutinity__ Scrutinity allows for __accountability__ > _It's okay to make mistakes, as long as one can detect them and that we can learn from them..._ ] .pull-right[ .img60[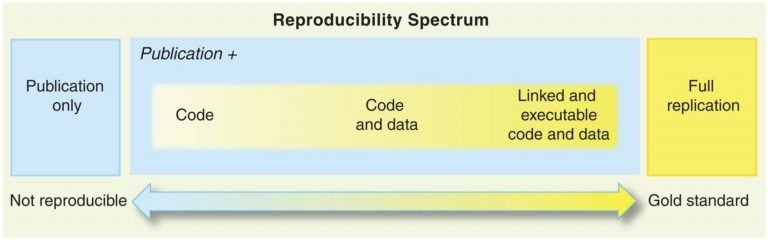] ] ??? Ethical principles into algorithmic design - would apply as well when designing household vulnerability scoring formula to inform humanitarian targeting - https://www.hum-dseg.org/sites/default/files/2020-10/Framework%20for%20the%20ethical%20use.pdf --- ## Productivity: getting things done quickly and safely! .pull-left[ <span style='font-size:30px;'>Automation through functions & scripts can help skipping __repetitive tasks__</span> > Tasks that involve recurrent __data manipulation__ are undertaken by teams... > .. but not everyone in the team needs to be a __geek/coder__! When enough investment can be made, __Graphical User Interface__ (GUI) can be developed for specific functions to ease the learning curve of new users while they are still in the process of building up their personal R skills. ] .pull-right[ .img130[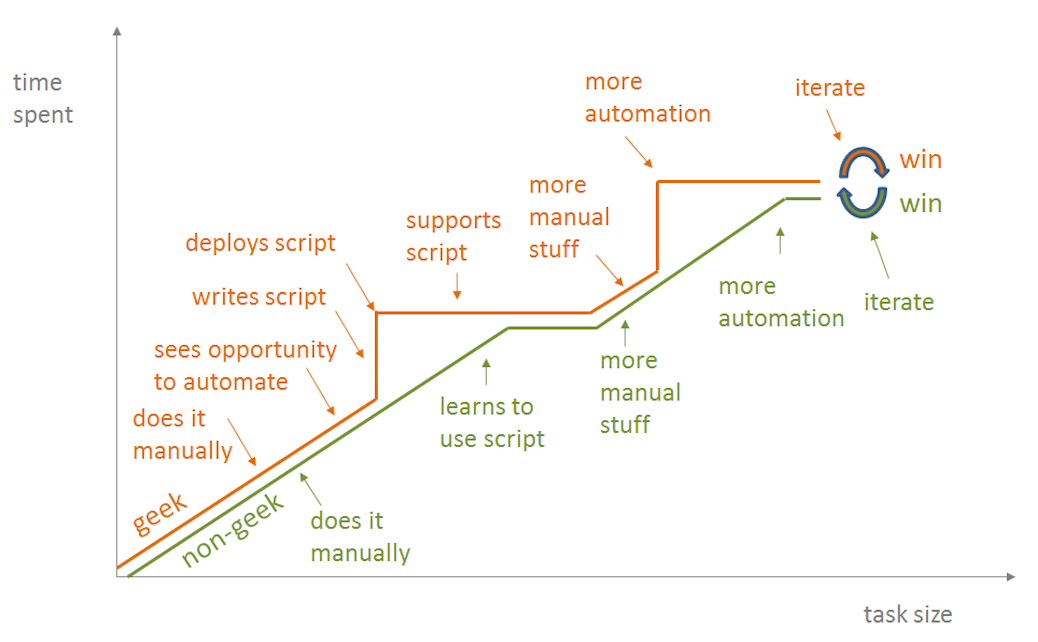] ] ??? https://blog.jonudell.net/2012/01/09/another-way-to-think-about-geeks-and-repetitive-tasks/ --- ## An R-Community geared towards learning .pull-left[ Which approach is the most appealing exercise among the 2 proposed aside?!! <span style='font-size:40px;'>Start from an end-product and __reverse engineer it__!</span> Eat the cake first! (then play with and change ingredients...) ] .pull-right[ .img[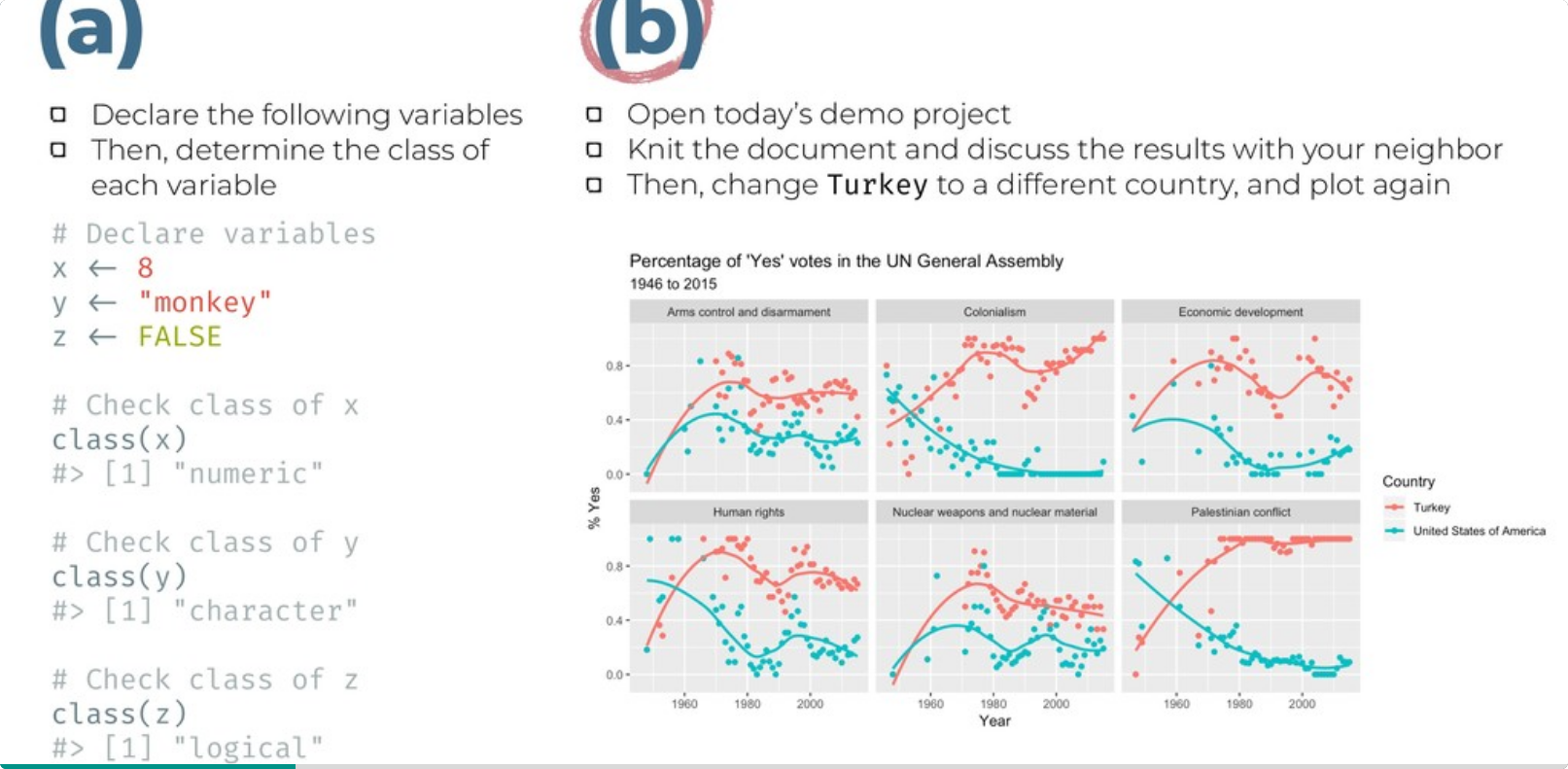] ] ??? https://speakerdeck.com/player/078592d490ae459790af7e77695fafcf?title=false&skipResize=true --- class: center, middle, inverse # Conditions for reproducibility. ### Sourcing data, documenting analysis, & packaging output --- ## Preparing data .pull-left[ Data Wrangling takes usually more than 80% of any data project time... Imagine if you need to rewind your analysis... Correct at any steps in the process and re-run all.. ] .pull-right[ .img[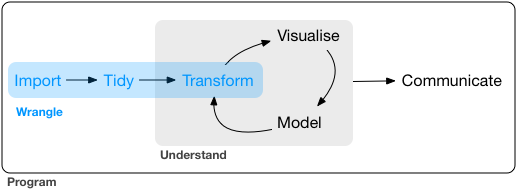] ] --- ## Documenting analysis .img[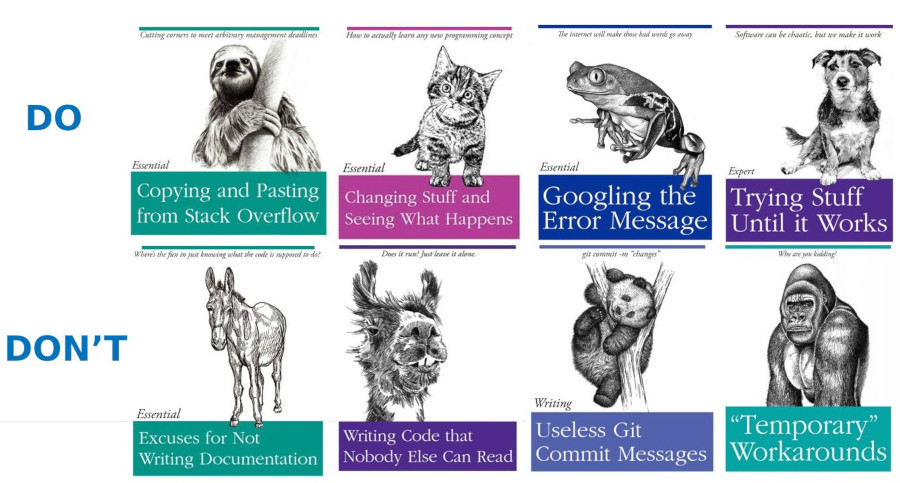] --- ## Packaging functions .pull-left[ Gradual automation * level 1: write a command * level 2: organize multiple command together in re-usable function * Level 3: organize multiple functions together in a package * Level 4: includes test data & Documentation * Level 5: <span style='font-size:30px;'>Unit testing, aka code review</span> * Level 6: <span style='font-size:30px;'>Graphical User Interface (GUI)</span> ] .pull-right[ .img75[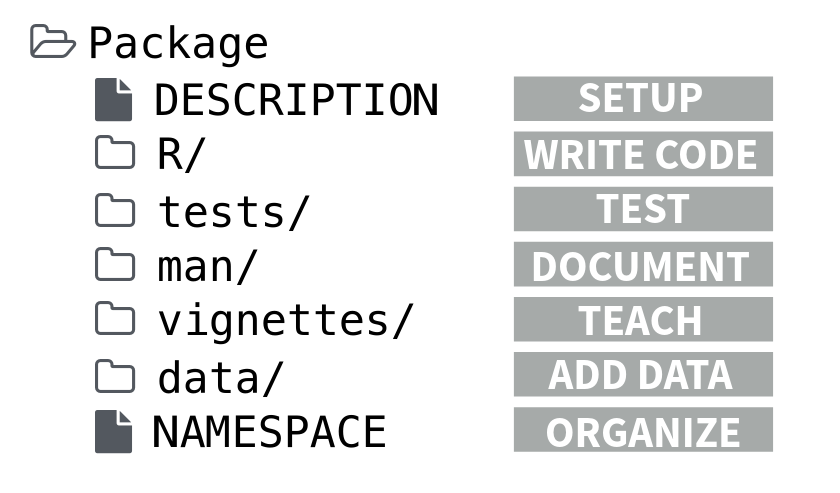] ] --- class: center, middle, inverse # Hands-on practice: a practical run-through based on Household survey dataset ### Crunching, Interpretation & Dissemination --- ## Step 1- Notebook for Automatic Data exploration, aka "crunching" .img75[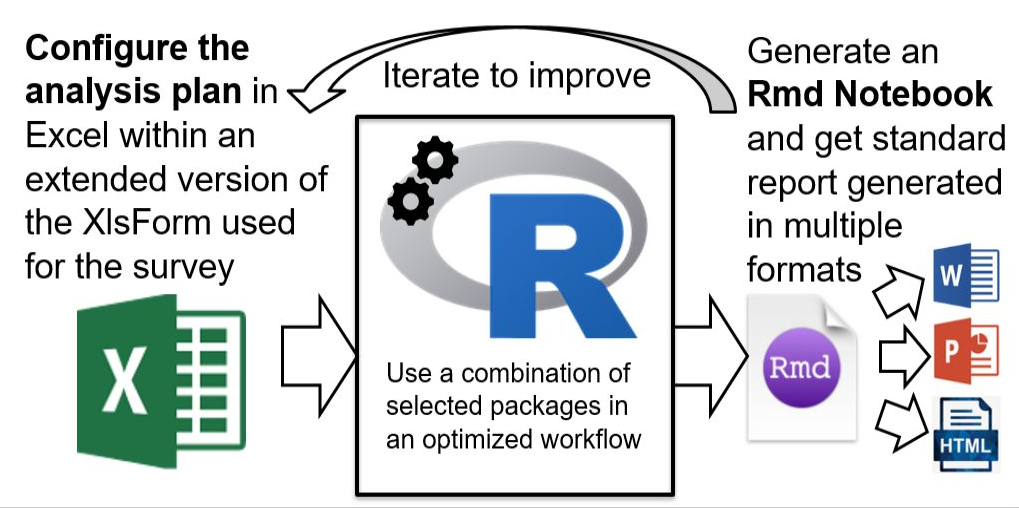] ??? A comparison of packages for Automated Exploratory Data Analysis https://arxiv.org/pdf/1904.02101.pdf https://github.com/mstaniak/autoEDA-resources One of the most significant disadvantages of PowerBI is that it is read-only. As a user, you cannot use charts/tables to make decisions and save them in a database directly. Also, PowerBI doesn’t have an accessible source code. You can only edit fields in WYSIWYG mode, which makes PowerBI easy to start but difficult to maintain. Having no source code makes it nearly impossible to have proper version control, automatically test logic, or collaborate on large projects. https://appsilon.com/powerbi-vs-r-shiny/ Pros of Power BI - Cross-filtering Pros of RStudio - - Visual editor for R Markdown documents - In-line code execution using blocks - Sophisticated statistical packages - Supports Rcpp, python and SQL - Can be themed - In-line graphing support - Latex support What is RStudio? An integrated development environment for R, with a console, syntax-highlighting editor that supports direct code execution. Publish and distribute data products across your organization. One button deployment of Shiny applications, R Markdown reports, Jupyter Notebooks, and more. Collections of R functions, data, and compiled code in a well-defined format. You can expand the types of analyses you do by adding packages. You can use R script with Power BI in several ways. The key ones include: - Data source – expand the range of available data sources, provide data processing logic at the import stage - Processing script – enrich data processing capabilities and engineering features - Visualization – extend the range of visualizations available in R and implement charts - As a part of Power BI visualization – gain the ability to build R-based visualizations and package them into ready-to-put boxes directly into Power BI. https://www.predicagroup.com/blog/visualizing-data-r-script-power-bi/ --- ## Step 2- Notebook for Data Insights documentation: Analysis Repo .pull-left[ > Insight: The capacity to gain an accurate and deep understanding of someone or something Not all charts will emulate need for interpretation - the data analyst need to gemerate the one that can create __debates__. Charts need to be __crafted__ - for instance use chart title framed as "opening question"... Insights arive when a multifunctional team is able to explain __unexpected patterns__, to challenge or revise __existing assumptions__, or to identify evidence to support __Call to action__. ] .pull-right[ .img[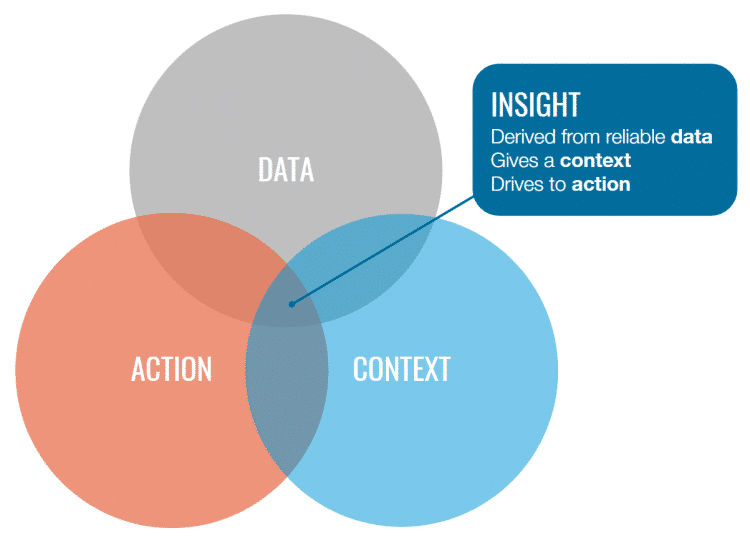] ] ??? https://www.ictworks.org/wp-content/uploads/2021/02/usaid-guide-artificial-intelligence.pdf https://bluemoondigital.co/our-blog/5-elements-storytelling-data/ Check the analysis repo: http://analysis.unhcr.org --- ## Step 3- Notebook to communicate with data: Microsite .pull-left[ <span style='font-size:30px;'>From __assumptions to evidence__ based statement</span> Data is to support Narrative - not the other way around! Leverage Art Data Storytelling to: * __Explain__, * __Enlighten__, * __Engage__ ] .pull-right[ .img75[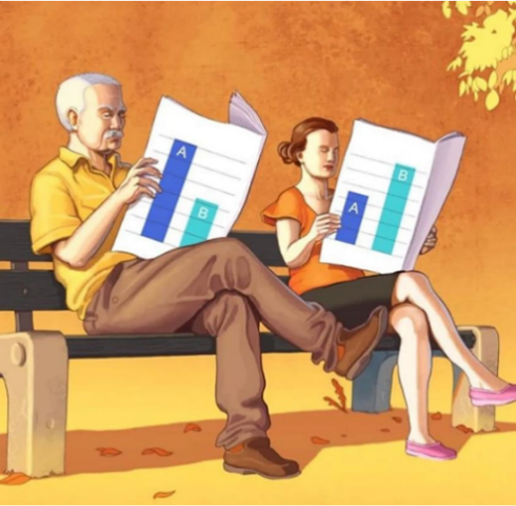] ] ??? See https://github.com/unhcr-americas/ageingonthemove/blob/main/README.md https://distill.pub/2020/communicating-with-interactive-articles/#applications-tab Research Dissemination Conducting novel research requires deep understanding and expertise in a specific area. Once achieved, researchers continue contributing new knowledge for future researchers to use and build upon. Over time, this consistent addition of new knowledge can build up, contributing to what some have called research debt. Not everyone is an expert in every field, and it can be easy to lose perspective and forget the bigger picture. Yet research should be understood by many. Interactive articles can be used to distill the latest progress in various research fields and make their methods and results accessible and understandable to a broader audience. Opportunities Engage and excite broader audience with latest research progress Remove research debt, onboard new researchers Make faster and clearer research progress Challenges No clear incentive structure for researchers Little funding for bespoke research dissemination and communication Not seen as a legitimate research contribution (e.g., to the field, or one's career) --- class: center, middle, inverse # Conclusion --- ## R in Humanitarian Context .pull-left[ <span style='font-size:50px;'>You are not alone</span> > More than 450 users from multiple organisation in the [humanitarian-useR-group](https://humanitarian-user-group.github.io/) > Around already ≈20 R champions within UNHCR vs more than 420 PowerBI Pro users > Try to start by using existing UNHCR packages and start from a project you can reproduce ] .pull-right[ .img[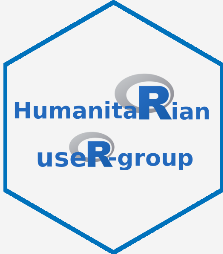] ] --- ## A call for Institutionalisation .pull-left[ Using Standard Multi Tier IT Standard Support model to enhance reproducible analysis... * Tier 4: Code Review & Quality Insurance / Contracted Company with global frame agreement * Tier 3: Internal package development / Internal R champions team (cost: one yearly Rdev meeting to incentivize contributing staff) * Tier 2: User induction & Advanced User Support / Global Data Service/DIMA (Data Science Team) * Tier 1: Basic User Troubleshooting / Global Service Desk (WIPRO according to Documented Scenario) * Tier 0: Self-support / Package documentation (maintained and improved on continuous basis) ] .pull-right[ .img75[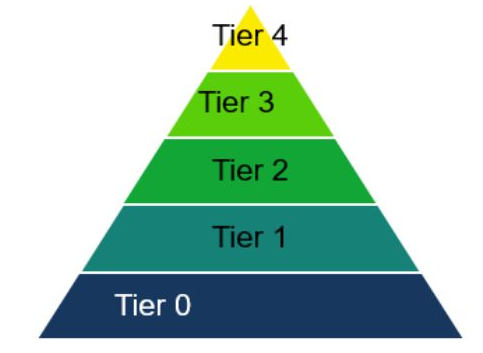] ] --- ## Your Opinion Count <span style='font-size:80px;'> Please [fill this survey](https://enketo.unhcr.org/single/bac3b19c91c4e5f22f4fa409bc618119) to share your opinion and thoughts on the topic presented here </span> Slides are available in [English](https://unhcr-americas.github.io/reproducibility/index.html) & [French](https://unhcr-americas.github.io/reproducibility/index_fr.html) - slides note can be displayed by pressing keyboard shortcut `p` - and in PDF ([English](https://unhcr-americas.github.io/reproducibility/index.pdf) & [French](https://unhcr-americas.github.io/reproducibility/index_fr.pdf)) within this [github repo](https://github.com/unhcr-americas/reproducibility)